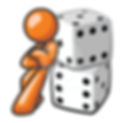
Permutation and Combination
It is important to understand the counting principles before jumping into the calculations of probability. Hence a brief of permutation and combination is given as under.
The permutation describes the arrangements and combination describes the groups. The mathematical representation of both counting principles can be given as per following description.

Few Important Results



Remark
If part of a work can be finished in 2 ways and the next part of the work can be finished in 3 ways. The total number of ways the complete work can be finished in 2 x 3 = 6 ways.

Remark: In case of repetition is allowed while counting the calculation differs. For Example, if three letters A, B and C are to be arranged without repetition the total possible ways are P (3, 3), while in case of repetition it is 3 x 3 x 3 = 27 possible ways are there.
Video Lecture Support: Following playlist provides the support for learning permutation and combinations via video lectures.
Introduction to Probability
Foreseeability and prediction play an important role in management. In business management planning for upcoming year’s production, purchases, expenses and else is done on the basis of prediction of demand or forecasting of demand as we call it. The forecasting on the basis of which key business decisions are made always involve an amount of uncertainty. For instance: the manager marketing research may predict the demand for next year as 10,000 units of a certain product but if a question is asked, how much he is certain about this prediction? he may answer it could be some percentage up or down. It is always better if the manager can make a prediction in such a way; “The sales for the particular product would be around 10,000 units during next financial year and I am 70% certain about this statement”. This allows the firms to make decisions under calculated risk. Therefore, “The numerical measurement of the uncertainty is called as Probability”.
In modern days in all fields of study and practice, theory of probability plays an important role in decision making. In business management the scope is very wide as it helps the organizations to calculate and manage risk as well.
For example:
In production, knowing the probability of failure of a machine helps the organization to keep the production going smoothly as timely maintenance or replacement can be ensured.
In macro level management, the probable projection of demand for the next year may help the firm in identifying their expenses, requirement of manpower, income and taxes well in advance.
In finance, knowing the probable income may help the firm in budgeting.
Some Important Definitions
Theory of probability becomes really easy to understand if it is studied under a systematic definition of routine actionable items. Hence, it becomes very important for a reader to be familiar with the terminology used in probability theory.
Random Experiment
An experiment that can result in any of the two or more possible outcomes if repeated under identical conditions is known as a random experiment. For example: tossing a coin is a random experiment that may result in one of the two possible outcomes i.e., head or tail. The outcome in this case may not be essentially same during the repetition of the experiment. Rolling of a die is another random experiment as the outcome of this random experiment may result in any of the six known outcomes.
Trail and Event
The number of times an experiment is repeated is known as the trail while the outcome of an event is known as an event. For example, if we toss a coin two times there are two trails of the random experiment, “tossing of a coin” conducted and with each trail two possible events are associated i.e., getting a head or getting a tail. Events can be simple or compound depending upon how they have been defined by the observer. For example: a random experiment of rolling a die may result into any of the six possible events i.e., 1, 2, 3, 4, 5, 6. The observer may define the events associated with random experiment of rolling a die as; EO: Getting and odd number and EE: Getting an event number. The events EO and EE are known as compound event and can be further decomposed into simple events such as EO1: Getting a 1, EO3: Getting a 3, EO5: Getting a 5, likewise, EE can be decomposed into simpler events as; EO2: Getting a 2, EO4: Getting a 4, EO6: Getting a 6. Hence, the events that can be decomposed into simpler events further are known as compound events else simple events.
Exhaustive Cases
The total number of possible outcomes of an experiment is known the set of exhaustive events or cases. For example: the set of exhaustive events or cases for a random experiment of tossing a fair coin can be given by {H, T}. Likewise, the exhaustive events or cases associated with a random experiment of throwing a fair die can be given by a set {1, 2, 3, 4, 5, 6}. Collection of all possible events is also known as a sample space.
Remark: Complement of an event A us defined as the collection of all the events apart from A.
Favorable Cases
The number of cases or events that associate with the desired outcome are known as favorable outcomes. For example, an observer may secure a win if the random experiment of rolling a die result in an odd number. This observer will secure the win if face of the die shows 1, 3 or 5. Hence, the number of favorable cases for the observer are three.
Mutually Exclusive Events
The events or cases than cannot occur simultaneously are known as mutually exclusive events or cases. For Instance: a random experiment cannot result into the events of getting a head and getting a tail at the same time. If a bag contains 2 orange and 3 green balls. Hence, the events of getting a head and getting a tail as an event of same toss are mutually exclusive. The random experiment of drawing a ball cannot result in getting an orange ball and one green ball at the same time. Hence, the events are mutually exclusive.
Equally Likely Events
If all the events associated with a random experiment have equal chance of occurrence, the events are known as equally likely events. For example, in a random experiment of rolling of die, all six numbers have an equal chance of occurrence and hence known as equally likely events. If an urn contains 2 orange and 4 green balls, then in a random experiment of drawing one ball from the urn can result into two not equally likely events i.e., getting an orange ball or getting a green ball. Here, the green balls are double in numbers and hence a green ball has a higher chance of coming out in a draw comparatively.
Independent and Dependent Event
If the happening of one event is impacting the event of other event the events are known as dependent events otherwise independent. For instance, if there are 4 red and three white balls in an urn. The random experiment is conducted in drawing two balls one by one without replacement. In this case the 2nd draw depends on the outcome of first event and hence the events are called as independent. Further if the ball drawn in first draw is replaced the outcome of second draw is not impacted by the first draw and the events are known as independent.
Remark: two events are known as complementary events if they are (i) collectively exhaustive and, (ii) mutually exclusive.
Probability Defined
In simple words, “the numerical measurement of uncertainty is known as Probability”. The numerical measurement oscillates between 0 and 1. When the numerical value of probability is 0, the event is known as impossible event and when the numerical measurement of probability results in 1, the event is known as certain or sure event. The probability is defined as per following methods






Baye's Theorem
Baye's theorem is developed with the idea that the probabilities revise themselves with the new information coming in. For example: an investor may have decided to invest in stocks of a particular construction company on Monday morning but the time he reaches the office, he finds the news that a newly constructed bridge of same company has collapsed early Monday morning and the investor now decides against his initial idea of investing in the particular firm.
This approach effectively uses the updating information to increase the effectiveness of decision making by suppressing the risk.
The probabilities before revision are called prior probabilities and the probabilities after revision are called posterior probabilities. The posterior probabilities are conditional probabilities.

Video Lectures
***