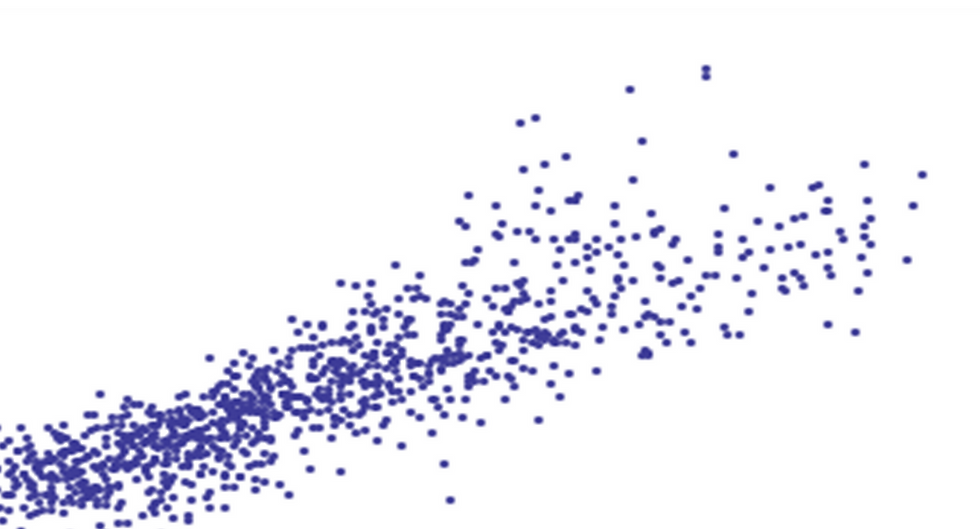
There are variables in the universe that vary together. In fact it will not be execration to say that all the variables except time are dependent on some variable or other. For Example: change in prices of steal may impact the cost of the cars, change in fuel prices may impact the cost of the travel, change in weather conditions may impact the sales of garments. Studying these relationships is very important for forecasting and measuring multi-dimensional impact of decision making. For example; before making changes in the tax slab, officials may try to forecast the multi-dimensional impact of it.
Above argument clearly indicates that, to study a relationship we shall have more than one variable under study. Hence, relationship of variables deals with bi-variate or multi-variate series not with the univariate series.
There are two phased to study relationship amongst the variables. First the relationship between the variables shall be established logically. For example; heavy rains in India may not impact the wheat production in China. If a researcher studies this relationship of variables i.e., rainfall in India and Production of wheat in China, it is ill logical. Hence, the in first step a logical relationship between the variables shall be studied. Sometimes the relationships are straight forward e.g., prosperity and happiness, oil prices and inflation, festival seasons and sales of sweets etc. at times the researcher has to find evidences for establishing a logical relationship. In second stage, the strength of these relationship is studied. For instance; change in oil prices may have a high impact on transportation cost, while a considerably low impact on happiness index.
The variables under consideration while studying correlation can be termed as independent or dependent variables depending upon their role. For Instance; when we are studying correlation between rainfall and production of wheat. The rainfall is independent and production of wheat is a dependent variable.
The sensitivity of correlation can be understood the amount of variation caused in dependent variable w.r.t. change in independent variable. For instance; a change in tax policy may have a high impact on savings of the people. This can be read as; the new tax scheme has caused a high variation in the saving amounts.
While studying the correlation one shall keep an eye on sufficiency of the data. There must be sufficient number of items in both the series for studying correlation. However, there is no defined limit on sufficiency but finding correlation on few entries may be highly misleading.
Statistics provides us facility to measure the strength of relationship using a tool called Correlation. Hence, correlation can be understood as a tool to measure the strength of a logically established relationship statistically or quantitatively. The strongest correlation gives the highest value of +1 or -1 while no correlation gives the value 0. Hence, the correlation value varies between - 1 and +1. Readers shall not confuse themselves as the lowest correlation value to be - 1. The + or - sign here represents the direction of change and will be explained later in the blog.
Higher value of correlation indicates a high sensitivity of the relationship. For instance; if the correlation between oil prices and cost of travel is 0.9, this indicates a tiny change in oil prices will create an immediate impact in travelling cost. While a low correlation value between amount of rainfall and patients visiting OPD say, 0.2 indicates that the large amount of rainfall will create a little variation in patients visiting the OPD i.e., the sensitivity is low.
Definitions of Correlation
Correlation measures the closeness of relationship between two variables, more exactly of the closeness of the linear relationship.
According to the words of Bodington ; “Whenever some definite connection exists between the two or more groups, classes or series or data there is said to be a correlation”.
Kinds of Correlation
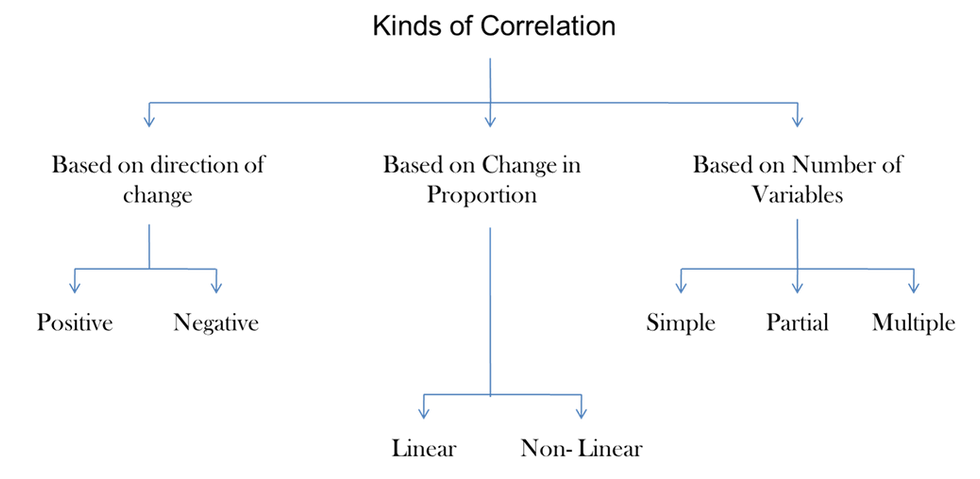
Positive and Negative Correlation
If two variables are moving in same direction, the correlation is termed as positive else negative. For instance; Rise in fuel prices may cause increase in transportation cost. Since both the variables in this example are moving in same direction i.e., one increases second also increases and vice-versa, the correlation is positive. While rise in fuel prices may reduce may impact negatively on number of travelers, this is called as negative correlation as increase in one caused decrease in other and vice-versa. A correlation value of - 0.8 indicates a high negative correlation, while a correlation value of +0.7 indicates a high positive correlation.
Linear and Non-linear Correlation
If there is a proportionate change caused in the dependent variable by independent the correlation is linear else non-linear. For Instance: If in at a certain workplace only two cups of complementary coffee is allowed to an employee during the day, then one unit change in employee strength in either direction will increase or decrease the consumption of coffee by two cups. Another example is; if 10% increase in salaries pushes the expenses of an organization by 12% every time, the change is called linear. However, most of the correlations that exist are non-linear in nature. For instance; hike in steal prices may impact the sales of motorbikes but not necessarily proportionately.
Simple, Partial and Multiple Correlation
There could be a possibility that there are more than two variables under study out of which one is dependent variable and rest are independent variables. For instance; Production of wheat may be impacted by Rainfall, Quality of Soil, Quality of Seed, Farming Method and other variables of such kind. In case we are studying only two variables i.e., one independent and one dependent, the study of correlation is known as single. When there are multiple variables under study but at a point of time only two variables are studied the study of variables is known as partial and if all the variables are studied together, the study is known as a multiple-correlation.
Measure of Correlation
The coefficient of correlation can be measured by any of three methods;
Scatter Plot
Karl Pearson's Coefficient of Correlation
Spearman's Rank Correlation Coefficient
Scatter Plot
This method is limited to simple correlation. This is a graphical method of studying correlation. The method involves plotting dependent variable on Y axis and independent variable on X axis. The method visually presents the high, low, positive or negative correlation. If all the points lie on a straight line having positive slope (i.e. rising line) the correlation is said to be perfect positive. In this case coefficient of correlation ‘r = + 1’.
If all the points lie on the line having negative slope the correlation is known as perfect negative. In this case coefficient of correlation ‘r = - 1. Following diagrams will provide a better clarity;
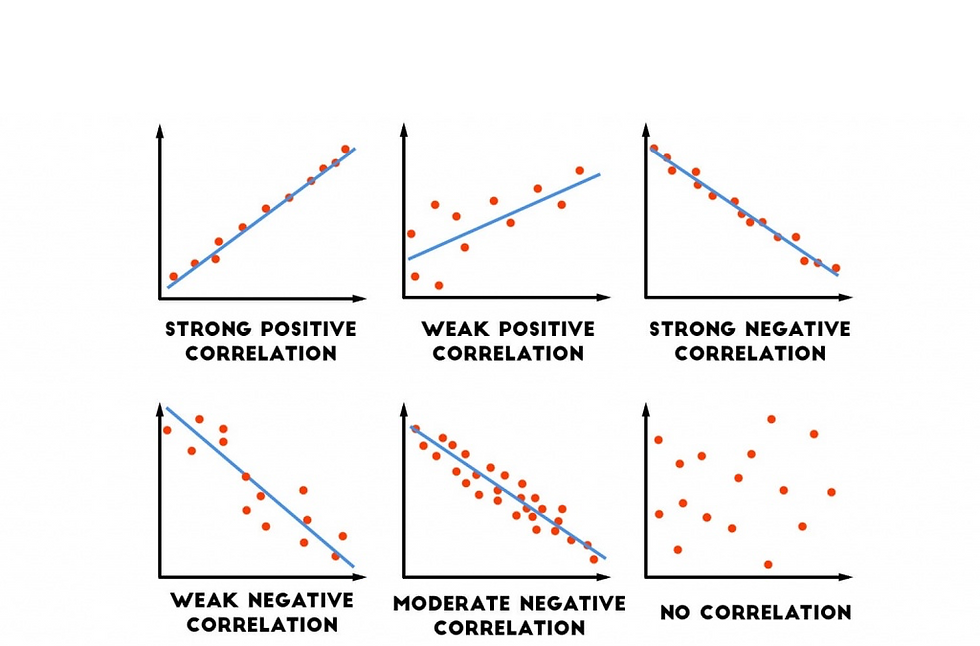
The difference between strong positive and weak or low positive graphs is that the deviation of the data points (dots) from the line is less in case one and high in case two. The same difference can be observed between strong negative and weal negative correlation. While, if there is no clear trend appears the correlation is considered as non-existent. Notice, that the direction of line shows positive or negative correlation. In positive correlation graphs the variables on X and Y axis are moving in same direction, while in negative correlation graphs the variables on X and Y axis are moving in opposite direction.
The scatter plot method gives only rough idea how the two variables are related. The methods gives an idea about the direction of correlation and also whether it is how or low. But this method does not give any quantitative measure of the degree or the extend of correlation. However, it is always advised to plot the data.
Karl Pearson's Coefficient of Correlation
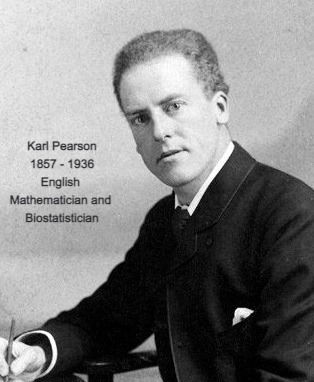
Is is a mathematical method of measuring the intensity or the magnitude of linear relationship between two variable series was suggested by Karl Pearson (1867 – 1936), a great British Biometrician and Statistician and by far the most widely used method in practice. Karl Pearson’s measure is known as Pearson’s correlation coefficient between two variables (series) X and Y, usually denoted by ‘r’, is a numerical measure of linear relationship between them.
Assumptions of Karl Pearson's Coefficient of Correlation
Karl Pearson’s coefficient of correlation as based on the following assumptions
Linear Relationship: In this method a linear relationship between two variables is assumed. In such case, the paired observations on the two variables plotted on a scatter – diagram cluster around a straight line.
Causal Relationship: In studying correlation, we expect a cause and effect relationship between the forces affecting the values in the two series.
For ungrouped data. Karl Pearson’s coefficient of correlation can be obtained by using any of the following three methods;
Actual Mean Method
Direct Method
Short – Cut Method
Actual Mean Method
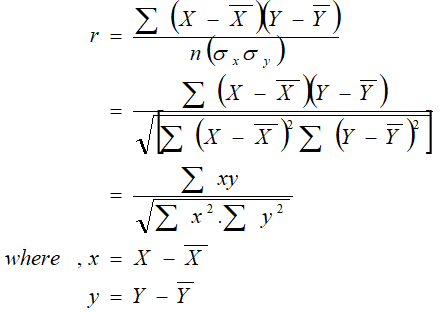
Direct Method
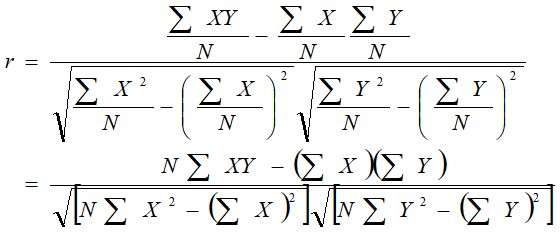
Short-cut Method
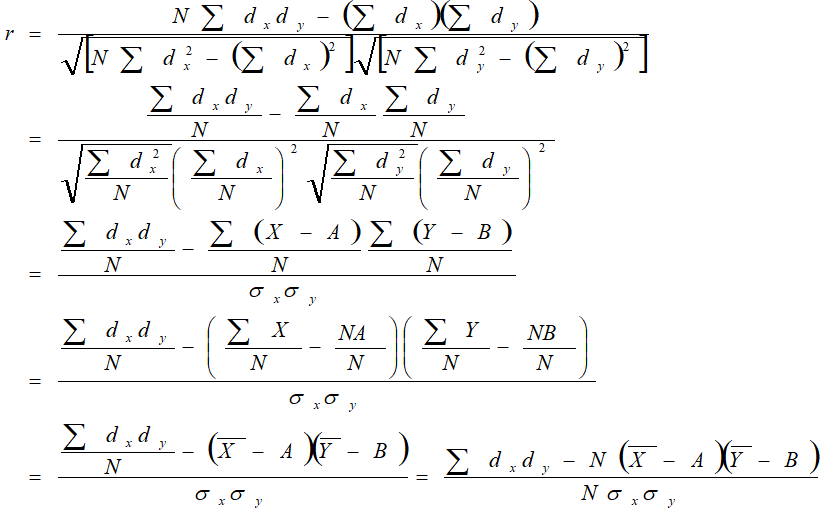
Spearman's Rank Correlation Coefficient
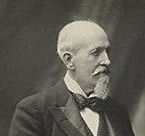
Rank Correlation Coefficient permits us to correlate two sets of positive of qualitative observations which are subject to ranking such as qualitative productivity ratings (poor, fair, good, very good, etc.) for a group of workers by two independent observers. This will also give an idea whether the two observers have common or different tastes likings in a particular attribute or characteristics. Ranks can be assigned either by two persons (called judges) to a single characteristics, say, beauty, honesty, intelligence, etc., or by a single person or two characteristics. When the marks are assigned by two persons to a single characteristics, the correlation is found between the opinion or tastes of the two persons. High positive correlation indicates that the two persons have the same taste in that characteristic. If two characteristics are judged by the same person, e.g., marks obtained in training and quantum of sales, then correlation is found between two characteristics.
To Calculate the Rank Correlation Coefficient
We first rank the two series say X’s and Y’s individually among themselves, giving rank 1 to the largest (or smallest) value, rank 2 to the second largest (second smallest) and so on in each series separately.
Find the differences ‘D’ of the corresponding Ranks of X and Y.
Sequence these differences and find the sum of the squares of these differences
Calculate rank correlation coefficient by using the formula
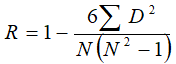
Where, ‘N’ denotes the number of paired values.
The above formula is applicable when no value in any of the two series is repeated. (Repeated values are known as tied values and are given the same Rank). When there are ties, we assign to each of the observations the mean of the ranks which they jointly occupy.
For Example:
If the third and fourth largest values of a variable are the same, we assign to each values, the rank = (3 + 4)/2 = 3.5 and if the fifth, sixth and seventh largest values of a variable are the same, we assign to each rank = (5 + 6 + 7)/3 = 6.
When some of the values are repeated and average ranks are assigned, the following formula is used to calculate rank correlation coefficient;

Where m = number of times a particular value is repeated. Repetition of values can be one series or both the series. Repetition can be in one value or more than one value.
Comments